Behavior Analysis and Data
Since the inception of behavior analysis to today, one constant has remained – an uncompromising commitment to data for the discovery, analysis, interpretation, and communication of behavior. The unearthing of the principles of reinforcement came from data exquisitely displayed on cumulative records. Across time, visual patterns of regularity emerged leading B.F. Skinner and his contemporaries to establish the science of behavior and set it a course to continue flourishing.
Eventually, the first generation of behavior analysts thought the principles working in the experimental chamber so well could help people. Many regard Ayllon and Michael’s (1959) publication as the first applied behavior analytic paper. The paper demonstrated how behavior analysis could reduce consistent, challenging behavior in psychiatric patients, such as hoarding, problems with self-feeding, and psychotic talk. Front and center, Ayllon and Michael’s data conclusively show changes such as an extinction response of “entering the nurses’ office.”
Fast forward to today and some 35,000 certified behavior analysts continue to spread the power of applied behavior analysis in areas ranging from Autism and Behavioral Gerontology to Sports and Fitness and University Teaching. However, with the rapid expansion of behavior analysis, the consumption, management, and utilization of data remain more than a tradition, but a disciplinary imperative woven within ethical guidelines from the Behavior Analyst Certification Board (BACB) and position statements from the Association of Behavior Analysis International (ABAI).
With the rush of new professionals entering the field, some unfortunate problems have arisen. Namely, some people not receiving a full appraisal of how to thoroughly infuse data into their practices. The following form mainstays of a comprehensive data engagement system in the application of the science of behavior.
1) Detection: The ability to detect a behavior breaks down to a simple process. For human observation, the behavior analyst identifies a target and then must successfully distinguish the occurrence and nonoccurrence of the target. Several options exist for identification of the target: a general label, an operational definition, and a pinpoint. Additionally, detection includes a choice between continuous and discontinuous observation and complete or incomplete observation schedules.
2) Collection: Collecting data focuses mainly on how the behavior analyst counts the behavior and what representation of collected quantities. Two main options exist, dimensional and dimensionless quantities. Also specific to data collection, the tools associated with counting and the subsequent records generated. A human observer can use devices (e.g., mechanical counters, apps, paper and pencil) or rely on their own count (e.g., count with fingers, mental counts). The behavior analyst will record the count in a paper or digital medium or have an unrecorded count.
3) Management: The management of data falls into two main categories, (1) activities surrounding the “goodness” of the data and (2) the physical management of the data. Data accuracy serves as one of the main components of data quality. When examining existing data, people will wonder how accurate the collected data represents how the actual events in nature transpired. Activities used in behavior analysis entail Interobserver Agreement (IOA) and Accuracy. Sometimes data management embraces Reliability or how often does an observer consistently count the same behavior when it occurs.
4) Analysis: The process of carefully inspecting data to discover new information, confirm or disconfirm intervention effectiveness, or monitor the course of behavior requires diverse techniques. First, the medium with which a behavior analyst analyzes data can range from subjective appraisal and viewing numeric data to visual and quantitative analysis. Even within visual analysis, the most popular technique for scrutinizing data, choices exist as to which lens the behavior analysis will view the data – linear graphs or ratio charts. Quantifying data offers an even deeper level of processing information and ascertaining its meaning.
5) Decision Making: The act of making decisions involves choosing a path of action from a number of alternatives. What informs someone’s decision hopefully results from a meticulous process detection, data collection, management, and analysis. Some people just make a gut decision or one based on intuition. Data provides insight and fine-grained, detailed, data-driven decisions. Furthermore, several strategies exist which behavior analysts can apply to both real time and historical data decisions.
Data Engagement
In a workplace, people have varied skills, experiences, and contingencies which all coalesce into how they engage data. The resources and choices one makes in regards to the previously described data practices and decision making can culminate in maximum efficiency and effectiveness in helping a client attain their individualized goals. On the other hand, flouting the best practices of measurement and decision making science will result in minimal impact or worse, clients with lingering or worsening behavior.
The following figure depicts profiles of 5 different types of measurement and decision making engagement practices.
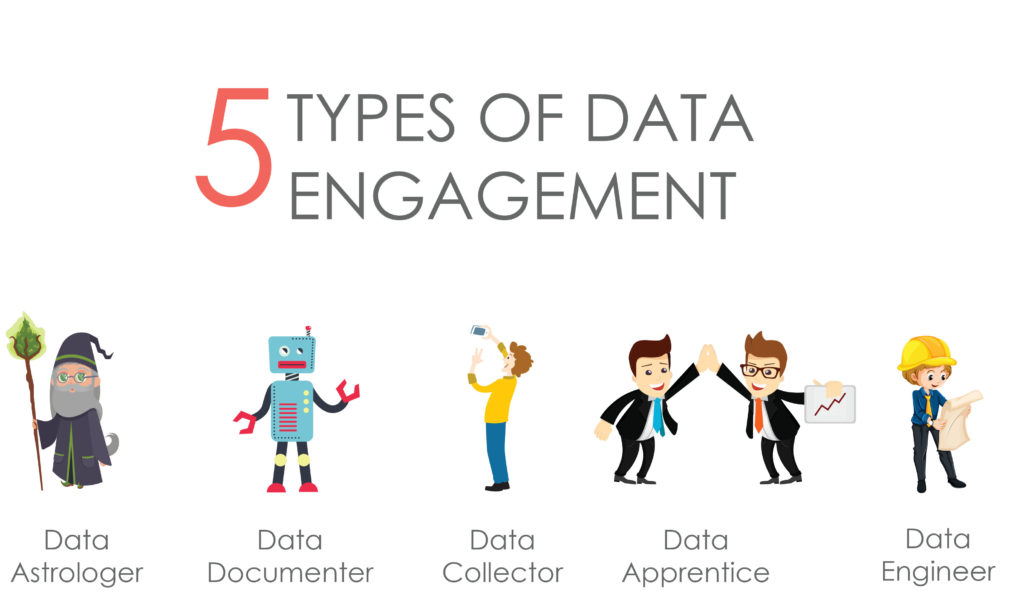
Figure 1. Five profiles of the different type of data engagement practices people can assume.
Data Astrologer: In the pseudoscience astrology, astrologers try and derive information about human behavior and events through the position of the stars and planets. According to astrologers, a person’s zodiac sign supposedly reveals what someone should do and watch for. No rational basis or evidence exists to support astrology, thus its inclusion as a pseudoscience.
Data astrologers violate every positive tactic of behavioral measurement and decision making conceived. Data astrologers eschew strong detection procedures in favor of using their subjective definitions of what they should notice. Data collection methods eschew counts of behaviors and instead reside “inside the person’s head.” In other words, a subject impression of how often a client behaves emerges without any objective count or physical record.
Managing data that resides within a person means no effective management strategies can manifest. And because all of the data has not become public, the analysis must also remain within the person. “Data concealment” opposes “data transparency” and best characterizes how a data astrologer manages and analyzes data.
All of the data measurement and management operations converge to render a data-informed decision. Data astrologers render decisions based on subjective, inconsistent, unverifiable hunches.
Data Documenter: Recordkeeping no doubt plays a critical role in every behavior analytic practice. Detecting behavior, counting the observations (or checking records in the case of using machines or devices to capture behavior), and annotating notes all must occur within a sound data analytic plan.
However, data documenters fashion the emergence of data as a “win.” Logging in data marks the arrival and presence of the all so important data. But a chronicle of data does no better than telling someone with strep throat that penicillin sits on a shelf at a pharmacy.
Many people understand the critical need to obtain data. Behavior analytic textbooks, behavior analytic supervisors, and even stakeholders and payors communicate and demand measurement, collection, and the subsequent analysis of data. But the analysis and decision making functions of data have not impacted data documenters. Without an analysis, insight will not manifest itself. And without insight, no credible means to take action and help a client can transpire.
Data Collector: Like the data documenter, data collectors get some of the overall measurement package correct. Data collectors may also emphasize the need for quality data and accessible storage systems. Some data collectors would even promote standard and ad hoc data reporting. A healthy respect for organizational measurement capability does make for a positive characteristic.
Nevertheless, data collectors stop at a vital stage, decision making. The accumulation and ease of use of data form a vital part of every organization or individual behavior analyst’s journey to fully executing visual, quantified and qualified information. Collectors value data for data’s sake as opposed to what data can enable – analysis, insight, and action. As an analogy, placing a street sign to warn people of danger and then no one pays attention to it wastes people’s time. Therefore, collecting data without acting on it will not lead to a meaningful impact in the life of a client.
Data Apprentice: Five major activities constitute data engagement: detection, collection, management, analysis, and decision making. Delving into each separate component brings with it potential pros and cons. For example, discontinuous versus continuous has ramifications as to the representativeness of the collected data.
The data apprentice finds themselves learning how to bring into play the best of the five ingredients of data engagement. Achieving a balance of not only measurement but analysis and decision making takes time, experience, and coaching.
Data Engineer: The quality of insights relies on the analysis which directly stems from the quality of the data. The “data up” process begins with having excellent detection and ends with strong recording. Next, solid data management assures readily available and transparent data. Different levels of review and checks and balances all appear in a strong data management system.
Data engineers also review data with standard visual displays, thereby reducing interpretative errors while at the same time bolstering pattern recognition. A thorough analysis ensures making use of all visual analytic tactics, as well as augmented quantified analysis. The findings lead to insights into how intervention affects behavior which facilitates smarter decisions.
Conclusion
High quality, fully-validated data comes from a hard-fought process of data detection and collection. When managed well, the data then provides a great focus for analysis and subsequent decision making. When everything comes together, behavior analysts can make smarter decisions and create efficiencies for their clients. Yet not everyone engages data as thoroughly and intensively as others. The foundation for creating and fostering data engineers lies in a multi-component system of using the best practices of data detection, collection, management, analysis, and decision making.
About the Author
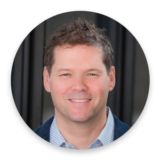
Dr. Rick Kubina, BCBA-D
CentralReach Director of Research
Richard M. Kubina Jr., Ph.D., BCBA-D is a Professor of Special Education at The Pennsylvania State University and teaches courses on methods for teaching reading, behavior analysis, and experimental design. Rick graduated from Youngstown State University where he had Steve Graf as an advisor and then received a Masters and Ph.D. from The Ohio State University under John Cooper. Rick conducts wide-ranging research in the area of Applied Behavior Analysis and Precision Teaching. He also served as the editor of the Journal of Precision Teaching and Celeration. Rick has dedicated his professional career to helping behavior change agents such as teachers, behavior analysts, and other helping professionals efficiently change behavior through effective teaching and measurement such as Precision Teaching. Rick co-founded a software called Chartlytics. Chartlytics merged with CentralReach where Rick has assumed the role of Director of Research. At CentralReach, Rick explores how technology can accelerate superior outcomes for all those seeking to engender professional and personal success.